Impact of AI and Machine Learning on Digital Advertising

Overview of AI and machine learning
AI, or Artificial Intelligence, refers to the system of human intelligence by machines, allowing them to perform tasks that typically require human intelligence, such as learning, problem-solving, and decision-making. Machine learning, a subset of AI, focuses on the development of algorithms that allow computers to learn from and make predictions or decisions based on data, without being clear programmed. In the context of digital advertising, AI and machine learning are revolutionizing the industry by enabling specific targeting, deep analytics, automated optimization, and enhanced customer insights, leading to more efficient and effective advertising campaigns.
Introduction to digital advertising
Digital advertising requires marketing efforts conducted through online channels, such as websites, social media platforms, search engines, and mobile apps, to reach and engage target audiences. It consists various formats, including display ads, video ads, sponsored content, and search engine marketing, to promote products, services, or brands. With its ability to target specific demographics, track performance metrics, and optimize campaigns in real-time, digital advertising offers unparalleled opportunities for businesses to reach their audience effectively in today’s increasingly digital world.
Exploring the transformative effects of AI and machine learning
AI and machine learning are reshaping the digital advertising world by enabling unprecedented levels of precision, personalization, and efficiency. These technologies provide power advertisers to analyze wider amounts of data, predict consumer behavior, and deliver highly targeted ads in real-time. By automating processes such as ad creation, placement, and optimization, AI-driven advertising campaigns can achieve better results while minimizing costs and maximizing ROI, revolutionizing how brands connect with their audiences in the digital realm.
Background of digital advertising
Digital advertising traces its roots back to the early 1990s with the launch of the first clickable banner ad by AT&T on HotWired.com. Throughout the 2000s, the industry witnessed rapid growth with the expansion of the internet and advancements in technology. Key milestones include the introduction of Google AdWords in 2000 and the rise of social media platforms like Facebook, which revolutionized targeted advertising. The evolution continues with the emergence of AI and machine learning, fundamentally changing how advertisers reach and engage with their audiences.
Traditional approaches and limitations
Statistics and data implement the rapid rise of voice search usage, highlighting its increasing prominence in the digital landscape. For instance, studies show that by 2022, voice-based shopping is projected to reach $40 billion in the United States alone. Additionally, research indicates that over 50% of all searches are expected to be voice-based by 2024. These figures underscore the growing reliance on voice search technology and its significant impact on consumer behavior and search trends.
1. Static Banner Ads
- Static banner ads were one of the earliest forms of digital advertising.
- Limitation: Lack of interactivity and engagement, leading to low click-through rates and effectiveness.
2. Manual Targeting
- Advertisers manually select audience demographics and interests for ad placement.
- Limitation: Limited precision and scalability, often resulting in ineffective targeting and wasted ad spend.
3. Cookie-Based Tracking
- Tracking user behavior through cookies to deliver targeted ads.
- Limitation: Reliance on third-party cookies, which are increasingly restricted due to privacy concerns, leading to challenges in accurate targeting and measurement.
4. Manual Ad Optimization
- Advertisers manually monitor and adjust ad campaigns based on performance metrics.
- Limitation: Time-consuming and resource-intensive, often leading to suboptimal campaign performance and missed opportunities for improvement.
5. Lack of Personalization
- Generic ad content delivered to broad audiences.
- Limitation: Reduced relevance and engagement, as ads fail to address individual preferences and interests.
6. Inability to Adapt in Real-Time
- Ads are static and do not adapt based on user behavior or changing market conditions.
- Limitation: Inability to capitalize on immediate opportunities or address shifting audience needs, resulting in missed opportunities for effective advertising.
7. Limited Measurement and Attribution
- Challenges in accurately measuring ad performance and attributing conversions to specific campaigns.
- Limitation: Difficulty in determining the ROI of advertising efforts and optimizing future campaigns based on actionable insights.
8. Siloed Data and Fragmented Campaign Management
- Data collected from various sources and platforms are often fragmented and not easily integrated for holistic campaign management.
- Limitation: Hindered ability to analyze and leverage data effectively for targeting, optimization, and reporting purposes.
Explanation of AI and Machine Learning
AI, or Artificial Intelligence, requires creating systems that can perform tasks that typically require human intelligence, such as learning, reasoning, and problem-solving. Machine learning is a subset of AI that focuses on developing algorithms capable of learning from data to make predictions or decisions without being explicitly programmed. It allows computers to recognize patterns, adjust behaviors, and improve performance over time, playing a crucial role in areas like natural language processing, image recognition, and predictive analytics.
Importance of data and algorithms in AI-driven advertising campaigns
1. Data-driven Decision Making
- Data serves as the foundation for AI algorithms to analyze and derive insights.
- Allows advertisers to make informed decisions based on audience behavior, preferences, and trends.
2. Audience Targeting and Segmentation
- Data enables precise audience targeting by analyzing demographic, behavioral, and contextual information.
- Algorithms optimize targeting strategies to reach specific audience segments with tailored ad content.
3. Predictive Analytics
- Historical and real-time data are used to train machine learning models for predictive analytics.
- Algorithms forecast future trends, consumer behavior, and campaign performance, enabling proactive optimization and decision-making.
4. Ad Personalization
- Data-driven insights inform personalized ad content creation and delivery.
- Algorithms dynamically adjust ad elements such as messaging, imagery, and placement to resonate with individual preferences and interests.
5. Real-time Optimization
- Continuous data analysis allows AI algorithms to optimize ad campaigns in real-time.
- Algorithms adjust bidding strategies, ad placements, and creative elements to maximize performance and ROI based on immediate feedback.
6. Fraud Detection and Prevention
- Data analytics and machine learning algorithms identify patterns indicative of ad fraud, such as click fraud and bot traffic.
- Enable proactive measures to mitigate fraud risks and maintain the integrity of advertising campaigns.
7. Performance Measurement and Attribution
- Data-driven attribution models analyze the contribution of various touchpoints to conversion.
- Algorithms accurately attribute conversions to specific ad campaigns, channels, or interactions, facilitating ROI analysis and optimization.
8. Continuous Learning and Improvement
- AI algorithms leverage data feedback loops to continuously learn and adapt to evolving trends and user behavior.
- Enable iterative optimization of advertising strategies, leading to improved campaign performance and effectiveness over time.
Role of AI in Personalization
The role of AI in personalization is essential, as it allows advertisers to create content and experiences to individual preferences and behaviors at scale. By analyzing large amounts of data, AI algorithms can segment audiences, predict user interests, and dynamically adjust ad elements such as messaging, imagery, and offers in real-time. This personalized approach enhances relevance, engagement, and ultimately drives higher conversion rates and customer satisfaction in digital advertising campaigns.
Benefits of personalized advertising for brands and consumers
Long-tail and conversational keywords are crucial in voice search optimization due to their alignment with natural language queries. Unlike traditional short-tail keywords, long-tail keywords cater to specific search intents, increasing the likelihood of matching user queries. Additionally, conversational keywords mirror how users speak, enhancing the relevance and effectiveness of content in capturing voice search traffic. Integrating these types of keywords ensures better visibility and engagement with voice search users, driving targeted traffic to your website.
1. For Brands
- Improved Targeting: Personalized advertising allows brands to target specific audience segments based on demographics, interests, and behaviors, increasing the relevance of their messages.
- Enhanced Engagement: Tailoring ad content to individual preferences boosts engagement rates, leading to higher click-through rates, conversion rates, and overall campaign performance.
- Increased ROI: By delivering more relevant ads to the right audience at the right time, personalized advertising maximizes return on investment by minimizing wasted ad spend and increasing conversion rates.
- Brand Loyalty and Trust: Personalized experiences foster a deeper connection between brands and consumers, leading to increased loyalty, trust, and long-term customer relationships.
2. For Consumers
- Relevant Content: Personalized advertising ensures consumers receive ads that are relevant to their interests, preferences, and needs, enhancing their overall browsing experience.
- Time Savings: By presenting consumers with products or services tailored to their preferences, personalized ads help streamline the decision-making process, saving time and effort.
- Improved User Experience: Personalization leads to more enjoyable and engaging user experiences, as consumers are more likely to interact with ads that resonate with their interests and needs.
- Discovery of New Products: Personalized advertising introduces consumers to products or services they may not have discovered otherwise, expanding their options and enriching their shopping experiences.
Machine learning for predictive analytics
Machine learning is instrumental in predictive analytics by leveraging historical and real-time data to predict future trends, behaviors, and outcomes. Algorithms analyze patterns and correlations within datasets to make accurate predictions and decisions, such as customer purchasing behavior, ad performance, and market trends. By continuously learning from new data inputs, machine learning models improve their predictive capabilities over time, enabling advertisers to make proactive decisions and optimize strategies for better campaign outcomes.
Improved ad relevance and engagement
1. Precise Audience Segmentation
- Predictive targeting algorithms analyze vast datasets to segment audiences based on demographics, behaviors, and interests.
- Enables advertisers to deliver tailored ads to specific audience segments, increasing relevance and engagement.
2. Dynamic Ad Content Optimization
- Predictive models anticipate individual user preferences and behaviors, allowing for dynamic adjustment of ad content in real-time.
- Advertisers can personalize messaging, imagery, and offers to match the predicted interests of each viewer, maximizing engagement.
3. Anticipating User Intent
- Predictive targeting algorithms forecast user intent based on historical data and contextual signals.
- Enables advertisers to anticipate user needs and deliver ads that align with their likely actions, resulting in higher relevance and engagement.
4. Adaptive Bid Strategies
- Predictive targeting informs bidding strategies by predicting the likelihood of conversion for different audience segments.
- Advertisers can allocate ad spend more efficiently by bidding higher for segments with a higher probability of conversion, optimizing ROI and engagement.
5. Continuous Learning and Optimization
- Predictive targeting models continuously learn from new data inputs and user interactions to refine predictions.
- Advertisers can iterate and optimize ad campaigns based on real-time insights, ensuring ongoing improvement in ad relevance and engagement.
Automated ad creation and placement
Automated ad creation and placement enhance AI-driven tools to streamline the process of generating and distributing ads across various digital channels. These systems use algorithms to dynamically create ad variations based on defined parameters, such as audience segments and campaign objectives. By automating tasks such as ad design, copywriting, and placement, advertisers can achieve greater efficiency, scale, and relevance in their advertising efforts while reducing manual workload and optimizing performance in real-time.
Optimization of ad performance using AI algorithms
1. Real-Time Data Analysis
- AI algorithms continuously analyze performance metrics, such as click-through rates and conversion rates, in real-time.
2. Automated A/B Testing
- AI conducts automated A/B testing to experiment with different ad variations and identify the most effective elements.
3. Dynamic Campaign Optimization
- AI adjusts ad parameters, such as bidding strategies and targeting criteria, dynamically based on performance insights and user behavior.
4. Predictive Analytics
- AI algorithms predict future trends and user behaviors, enabling proactive optimization strategies to anticipate changes in ad performance.
5. Adaptive Learning
- AI models learn from past campaign data and user interactions to iteratively improve ad performance over time, adapting to changing market conditions and audience preferences.
AI-driven data analysis for customer insights
AI-driven data analysis allows comprehensive customer insights by processing vast amounts of data to identify patterns, trends, and correlations. These insights include demographic information, purchasing behavior, browsing history, and engagement metrics. By using advanced algorithms, AI uncovers actionable insights that inform marketing strategies, product development, and customer relationship management. This approach facilitates personalized interactions, targeted campaigns, and improved customer experiences, covering enhanced satisfaction, loyalty, and business growth.
Understanding consumer behavior through machine learning
1. Data Collection and Integration
- Gathering data from various sources such as website interactions, purchase history, and social media activity.
- Integrating diverse datasets to create a comprehensive view of consumer behavior.
2. Pattern Recognition
- Machine learning algorithms analyze data to identify patterns, trends, and correlations in consumer behavior.
- Recognizing recurring behaviors such as browsing habits, purchase preferences, and engagement levels.
3. Predictive Modeling
- Utilizing historical data to build predictive models that anticipate future consumer actions and preferences.
- Predicting outcomes such as purchasing decisions, product interests, and churn likelihood.
4. Segmentation and Personalization
- Segmenting consumers into distinct groups based on their behavior, preferences, and characteristics.
- Personalizing marketing strategies, product recommendations, and content to match the needs of each segment.
5. Real-Time Adaptation
- Continuously updating models based on new data inputs and evolving consumer behavior.
- Adapting strategies and offerings in real-time to meet changing consumer preferences and market dynamics.
6. Feedback Loop Optimization
- Incorporating feedback from consumer interactions to refine models and improve predictive accuracy.
- Iteratively optimizing machine learning algorithms to better understand and anticipate consumer behavior over time.
Predictions for the future of AI in digital advertising
- Hyper-Personalization: AI will enable even more precise targeting and personalized ad experiences, catering to individual preferences and behaviors with unprecedented accuracy.
- Enhanced Automation: AI-driven automation will streamline campaign management processes further, from ad creation to optimization, freeing up marketers to focus on strategy and creativity.
- Integration with Emerging Technologies: AI will increasingly integrate with technologies like augmented reality (AR) and virtual reality (VR), revolutionizing immersive advertising experiences and blurring the lines between digital and physical worlds.
- Ethical Considerations: As AI becomes more pervasive in advertising, there will be an increased focus on addressing ethical concerns around data privacy, transparency, and algorithmic biases, shaping the regulatory landscape and industry practices.
In conclusion, the impact of AI and machine learning on digital advertising is vast and far-reaching. These technologies have revolutionized the industry by enabling unprecedented levels of actions, personalization, and efficiency. From predictive analytics and targeted advertising to automated optimization and increased customer insights, AI-driven strategies have transformed how brands connect with their audiences in the digital world. While the future holds even greater potential for innovation and integration with emerging technologies, it’s important to navigate the ethical considerations and regulatory challenges to ensure responsible and effective use of AI in design the future of digital advertising.
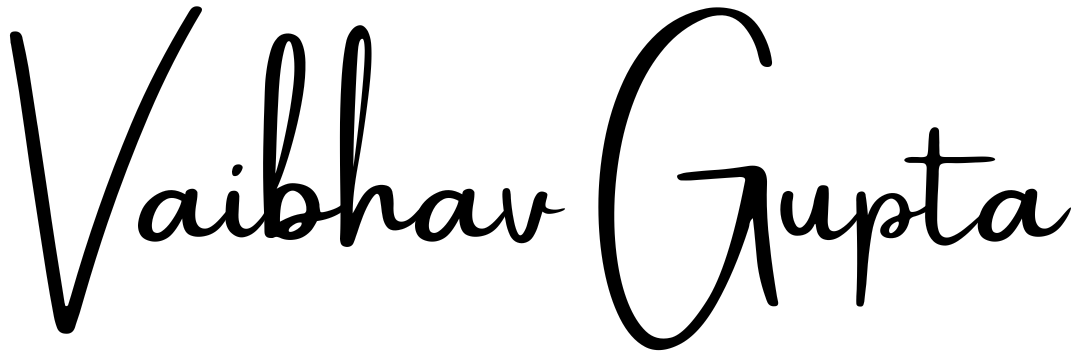